From logic gates to neural states
My presentation at HUMM PhD Student Conference at Tallinn University. Keeping it succinct as I hope to get this published as a paper
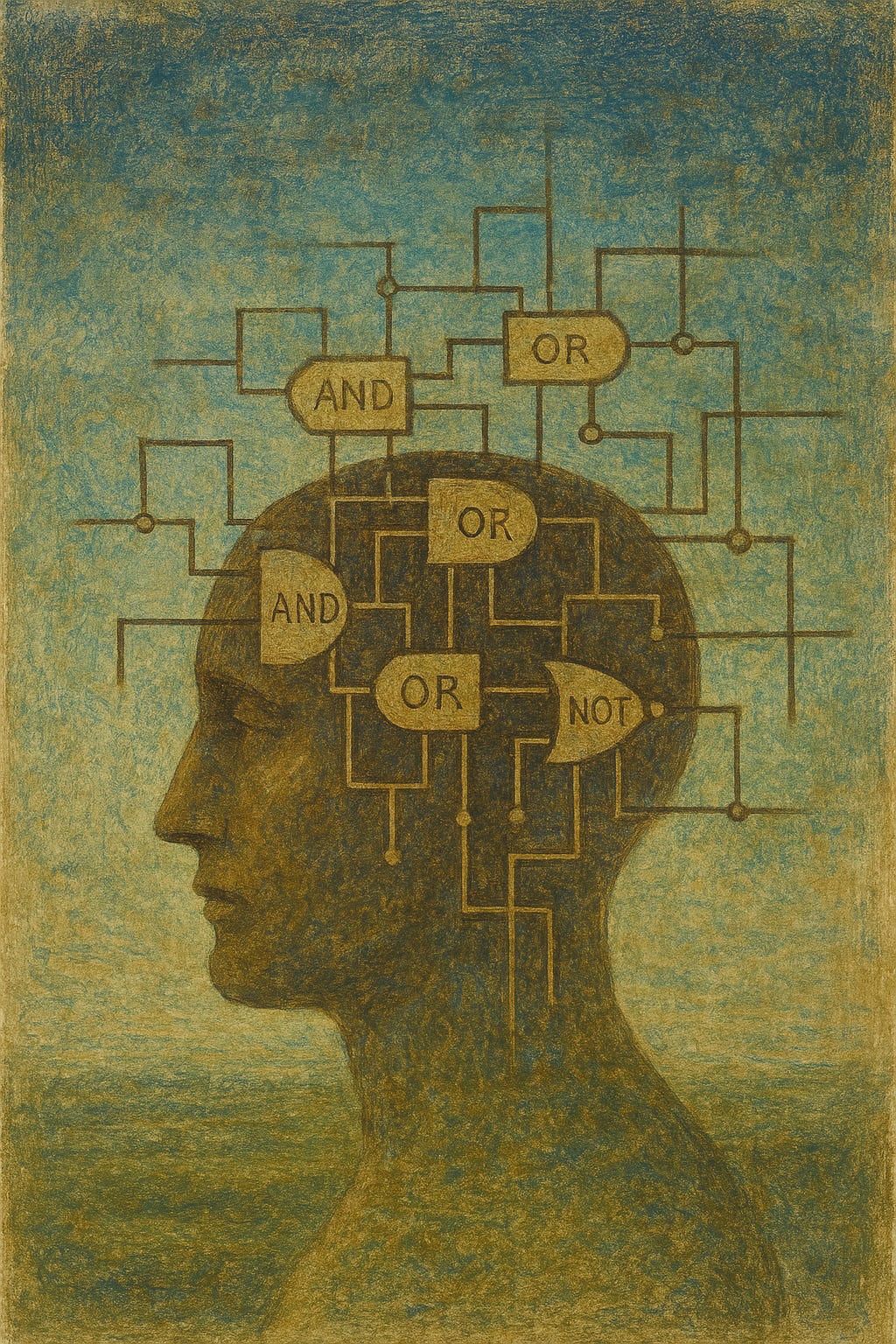
AI has revolutionized our understanding of cognition, but paradoxically, it has also highlighted the limits of computational metaphors when applied to the human mind. As AI evolves—from symbolic systems to neural networks, deep learning architectures, and large language models—it exposes insights into human cognition that machines cannot replicate. This post is a summary of my presentation at HUMM PhD Student Conference at Tallinn University, where I explored these paradoxes and argued why true intelligence is more than computation.
Four Paradoxes of AI Evolution
AI’s development is marked by four distinct paradigms, each revealing unique limitations:
Symbolic AI: Clumsy Grandmasters
Symbolic AI systems excel in formal domains like theorem proving or structured problem-solving but fail miserably in unpredictable, everyday contexts. Early systems like SHRDLU could simulate spatial reasoning within predefined rules but collapsed when faced with ambiguity or complexity outside their programmed environment. This era demonstrated that rigid rule-based systems lack the adaptability essential for real-world cognition.Neural Networks: Conceptless Geniuses
Neural networks introduced flexibility and the ability to identify patterns in vast datasets, from handwriting recognition to speech transcription. However, they lack conceptual understanding—operating as statistical tools rather than cognitive agents. While these networks mimic human-like outputs, their processes remain opaque and devoid of semantic grounding.Deep Learning: Mysterious Giants
Deep learning architectures scaled neural networks to unprecedented sizes, enabling breakthroughs in computer vision, natural language processing, and autonomous systems. Yet their complexity makes them inscrutable even to their creators. Despite their power, deep learning models are fragile, prone to errors from minor perturbations, and lack moral agency or self-awareness.Large Language Models: Oversaturated Prophets
Models like ChatGPT can generate fluent text indistinguishable from human writing but struggle with factual accuracy, coherence, and semantic depth. They process language as statistical patterns rather than meaningful communication, highlighting the gap between linguistic fluency and genuine understanding.
Why Cognition Is Not Computational
Each AI paradigm underscores a fundamental truth: human cognition cannot be reduced to computational operations. Unlike machines:
Human intelligence is embodied: We navigate the world through sensorimotor experiences tied to our physical presence.
Cognition is socially embedded: Our minds are shaped by cultural practices and interpersonal interactions.
Moral agency is intrinsic: Humans deliberate and reflect on ethical choices; machines merely execute predefined tasks.
Reflective capabilities are unique: We possess the ability to question our own thoughts and decisions—a trait absent in AI systems.
These qualities make human cognition inherently situated and dynamic, resisting simplistic computational analogies.
Implications for AI Development
As AI systems integrate into critical domains like healthcare, law, and governance, their limitations raise ethical concerns:
Transparency: Deep learning models operate as "black boxes," making it difficult to understand or trust their decision-making processes.
Accountability: Without moral agency, who bears responsibility for AI-driven errors?
Cultural alignment: Machines lack lived experience and emotional context, making them ill-equipped to navigate complex social dynamics.
To address these challenges, some AI researchers propose hybrid approaches combining symbolic reasoning with neural networks (neurosymbolic AI) or embedding AI into sensorimotor feedback loops for embodied intelligence. These frameworks aim to bridge the gap between computational efficiency and meaningful cognition.
AI’s paradoxes reveal that human cognition transcends computation. Minds are not machines—they are embodied, cultural, moral entities capable of reflection and growth. As we design increasingly sophisticated AI systems, we must ensure they augment rather than constrain our collective intelligence. By embracing the complexity of human cognition, we can guide AI toward ethical integration into society—enhancing our autonomy rather than undermining it.
This journey is not just about building smarter machines; it’s about understanding what it means to be truly intelligent—and deeply human.
March 2025